On the Lovász theta function and some variants
Galli, Laura and Letchford, Adam Nicholas
(2017)
On the Lovász theta function and some variants.
Discrete Optimization, 25.
pp. 159-174.
ISSN 1572-5286
![[thumbnail of theta-function]](https://eprints.lancs.ac.uk/85914/1.hassmallThumbnailVersion/theta_function.pdf)
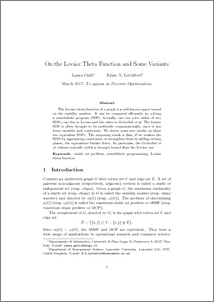
Preview
PDF (theta-function)
theta_function.pdf
- Accepted Version
Available under License Creative Commons Attribution-NonCommercial-NoDerivs.
Download (362kB)
Abstract
The Lovász theta function of a graph is a well-known upper bound on the stability number. It can be computed efficiently by solving a semidefinite program (SDP). Actually, one can solve either of two SDPs, one due to Lovász and the other to Gr�ötschel et al. The former SDP is often thought to be preferable computationally, since it has fewer variables and constraints. We derive some new results on these two equivalent SDPs. The surprising result is that, if we weaken the SDPs by aggregating constraints, or strengthen them by adding cutting planes, the equivalence breaks down. In particular, the Gr�ötschel et al. scheme typically yields a stronger bound than the Lovász one.
Item Type:
Journal Article
Journal or Publication Title:
Discrete Optimization
Additional Information:
This is the author’s version of a work that was accepted for publication in Discrete Optimization. Changes resulting from the publishing process, such as peer review, editing, corrections, structural formatting, and other quality control mechanisms may not be reflected in this document. Changes may have been made to this work since it was submitted for publication. A definitive version was subsequently published in Discrete Optimization, 25, 2017 DOI: 10.1016/d.disopt.2017.04.001
Uncontrolled Keywords:
/dk/atira/pure/subjectarea/asjc/1700/1703
Subjects:
?? combinatorial optimisationstable set problemsemidefinite programmingcomputational theory and mathematicstheoretical computer scienceapplied mathematicsdiscipline-based research ??
Deposited On:
13 Apr 2017 12:16
Last Modified:
15 Jul 2024 16:55